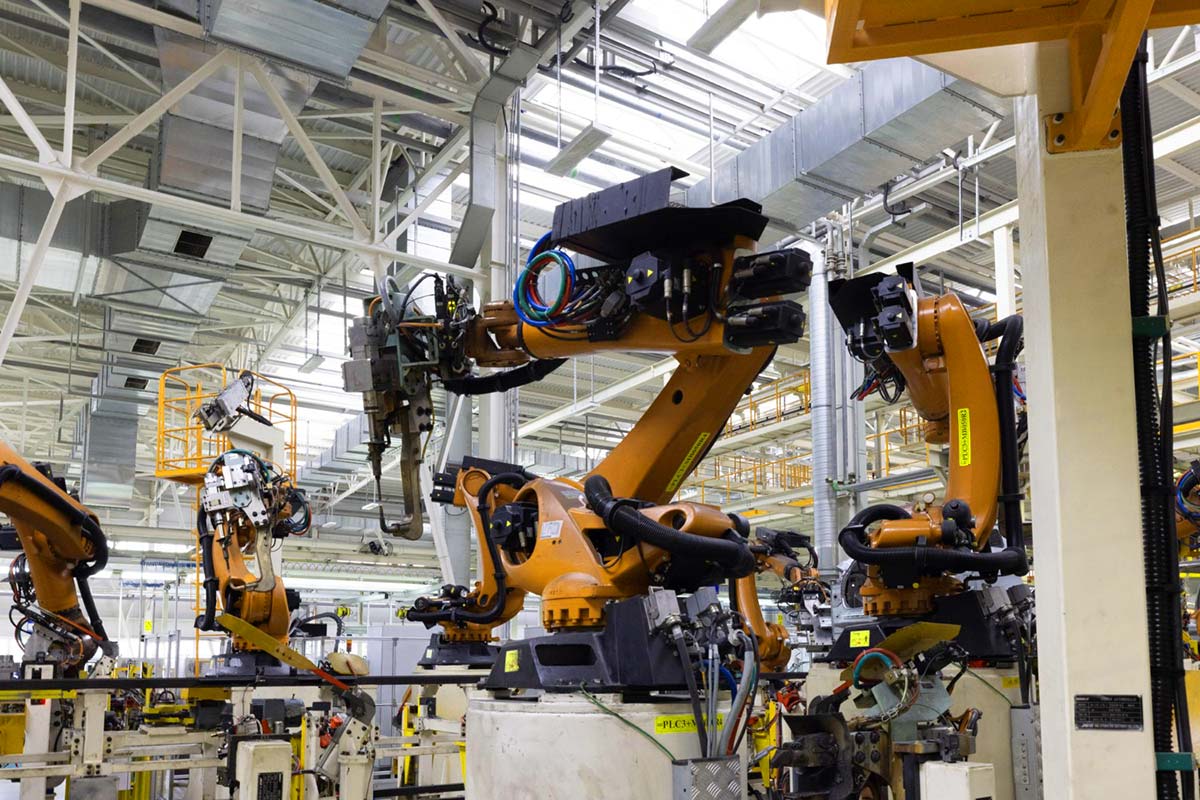
As businesses increasingly integrate AI/ML into their operations, managing complex ML workflows effectively becomes a pressing challenge. From data preparation and model training to deployment and ongoing monitoring, inefficiencies can lead to delays, increased costs, and inconsistent results.
Machine Learning Operations (MLOps) provides a structured approach to overcoming these hurdles. It unifies ML development with deployment and operations, ensuring that models move seamlessly from experimentation to production. By implementing MLOps, organizations can automate key processes, enhance collaboration between teams, and optimize infrastructure for scalability.
Understanding MLOps and Its Importance
MLOps functions as the backbone of successful AI implementation by integrating automation, standardization, and governance into ML workflows. It provides a framework that supports model development, validation, deployment, and maintenance, reducing friction between data scientists, engineers, and operations teams.
Without MLOps, organizations may struggle with inefficient workflows, unreliable model performance, and difficulties in scaling AI initiatives. It also helps maintain regulatory compliance, ensuring that AI models are fair, explainable, and free from biases. Moreover, MLOps aligns ML assets with the broader software development lifecycle, incorporating version control, continuous integration, and deployment best practices.
Core Principles of MLOps
MLOps are key principles that drive efficiency and reliability. Version control ensures traceability and reproducibility of ML assets, allowing teams to track changes in training data, code, and models. This approach enables organizations to roll back to previous versions if necessary and fosters better collaboration among stakeholders.
Automation is another crucial aspect, streamlining processes such as data ingestion, preprocessing, model training, validation, and deployment. Automated workflows eliminate manual errors, accelerate development, and support scalable AI initiatives. By leveraging triggers such as data changes, code updates, or performance monitoring alerts, organizations can ensure models remain up to date and effective.
The concept of continuous integration, delivery, training, and monitoring further enhances MLOps implementations. These interconnected activities ensure that new code, models, and datasets are rigorously tested and deployed with minimal disruption. Continuous monitoring helps detect model drift, performance degradation, and emerging biases, allowing timely adjustments to maintain accuracy and relevance.
Effective model governance is also essential. Beyond securing access to ML models and infrastructure, governance frameworks support fairness, compliance, and accountability in AI deployment. Clear documentation, stakeholder collaboration, and structured validation processes help mitigate risks and improve trust in AI-driven decision-making.
The Business Benefits of MLOps
Organizations adopting MLOps can expect significant improvements in AI efficiency and impact. By automating ML workflows, teams can reduce deployment times and accelerate time-to-market for AI-driven solutions. This agility enables businesses to stay competitive in rapidly evolving markets.
Productivity also receives a substantial boost. Standardized practices allow ML teams to collaborate more effectively, experiment faster, and repurpose components across different projects. Engineering teams can implement modular, reusable code, ensuring consistency and reducing redundancies across ML pipelines.
Beyond productivity gains, MLOps enhances scalability. By integrating models with CI/CD pipelines and maintaining structured deployment processes, businesses can manage multiple models simultaneously without performance degradation. This structured approach reduces operational costs and optimizes computing resources, making AI more cost-effective.
Additionally, a well-implemented MLOps strategy improves model performance and compliance. Continuous monitoring, bias detection, and governance frameworks help ensure AI models remain fair, accurate, and aligned with ethical standards. As regulations surrounding AI continue to evolve, MLOps provides a foundation for transparency and accountability.
Implementing MLOps at Different Maturity Levels
Organizations can adopt MLOps at varying levels, depending on their automation maturity and operational requirements:
Basic Level: Manual workflows dominate, with data preparation, model training, and deployment relying heavily on human intervention. This stage often lacks structured monitoring and versioning, making scalability difficult.
Intermediate Level: Automation begins to take shape. Training pipelines are automated, and CI/CD integration facilitates seamless deployment. Collaboration between data scientists and engineers strengthens, leading to more efficient workflows and improved model reproducibility.
Advanced Level: Full automation is achieved. Multiple ML pipelines run in parallel, supporting frequent model updates and real-time monitoring. AI-driven organizations leverage orchestrators, model registries, and governance frameworks to maintain control over AI deployments.
The Future of MLOps
As AI adoption continues to expand, MLOps is evolving to support emerging trends and challenges. Low-code and no-code platforms are making AI more accessible, enabling non-technical users to build and deploy models with minimal coding. These advancements reduce the barrier to entry for organizations looking to integrate AI into their operations.
Generative AI is also reshaping MLOps implementations. With the rise of large-scale language models, organizations require more sophisticated monitoring, fine-tuning, and deployment strategies. MLOps is adapting to manage these complex models efficiently while ensuring ethical AI practices.
Governance and ethical considerations are becoming more critical than ever. As regulations surrounding AI tighten, businesses must focus on bias detection, explainability, and fairness. MLOps provides a structured approach to addressing these concerns, ensuring AI models meet ethical and legal requirements.
Frequently Asked Questions (FAQs)
What is MLOps and why is it important?
MLOps is a set of practices that integrate machine learning model development with operational processes, ensuring efficiency, scalability, and governance in AI deployment.
How does MLOps improve AI scalability?
MLOps enables automation, continuous monitoring, and CI/CD integration, allowing businesses to manage multiple AI models efficiently without performance degradation.
What are the key principles of MLOps?
MLOps is built on version control, automation, continuous integration and delivery, governance, and monitoring, ensuring reliable and reproducible ML workflows.
How does MLOps help with AI model compliance and governance?
MLOps enforces security, bias detection, ethical AI guidelines, and structured validation processes to ensure compliance with industry regulations and fairness standards.
What industries benefit the most from MLOps?
Industries such as finance, healthcare, retail, and manufacturing leverage MLOps to improve AI-driven decision-making, automate workflows, and enhance customer experiences.
Conclusion
MLOps is a fundamental component of successful AI implementation, offering a structured approach to managing ML workflows. By integrating automation, governance, and continuous monitoring, organizations can accelerate model deployment, improve productivity, and optimize AI scalability.
As AI technologies continue to evolve, adopting MLOps is no longer an option—it’s a necessity. Businesses that embrace MLOps will be better positioned to navigate the complexities of AI deployment, ensuring their models remain effective, ethical, and aligned with business goals.
About NorthBay Solutions
NorthBay Solutions is a leading provider of cutting-edge technology solutions, specializing in Generative AI, Cloud Migration, ML/AI, Data Lakes and Analytics, and Managed Services. As an AWS Premier Partner, we leverage the power of the cloud to deliver innovative and scalable solutions to clients across various industries, including Healthcare, Fintech, Logistics, Manufacturing, Retail, and Education.
Our commitment to AWS extends to our partnerships with industry-leading companies like CloudRail-IIOT, RiverMeadow, and Snowflake. These collaborations enable us to offer comprehensive and tailored solutions that seamlessly integrate with AWS services, providing our clients with the best possible value and flexibility.
With a global footprint spanning the NAMER (US & Canada), MEA (Kuwait, Qatar, UAE, KSA & Africa), Turkey, APAC (including Indonesia, Singapore, and Hong Kong), NorthBay Solutions is committed to providing exceptional service and support to businesses worldwide.